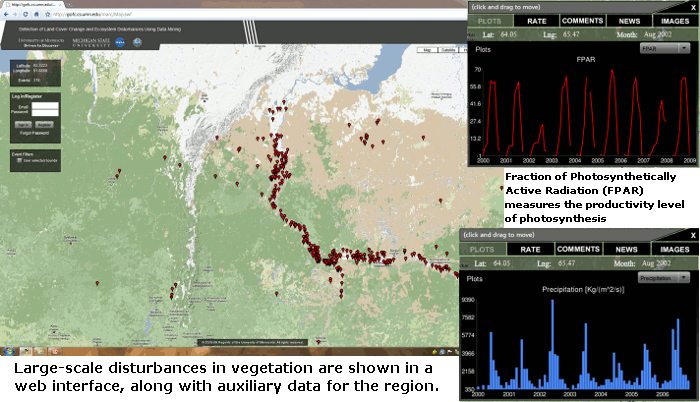
Climate change has become the defining issue of our era. There is an
increasing consensus that greenhouse gas emissions resulting from the
use of fossil fuels as well as human-induced changes to the ecosystem
(e.g. deforestation) are the cause of global warming, which in turn,
can have dramatic consequences such as increased occurrence of extreme
weather events, shocks in food and water supplies, rising sea
levels. This has created an urgent need to improve our ability to
answer questions such as: (i) what is the impact of global warming on
the frequency, intensity and duration of extreme events such as heat
waves, droughts, floods, hurricanes, large scale forest fires; (ii)
what is the impact of deforestation and other land cover changes on
the global carbon cycle; (iii) what is the relationship of crop prices
to deforestation dynamics and greenhouse gas emissions. There is a
significant interest in the ability to answer such questions from a
large community that includes climate and environmental scientists,
policy makers and just as importantly, the society at large. Data sets
that can help us answer such questions are becoming increasingly
available from remote sensors like satellites and weather radars, or
from in situ sensors and sensor networks, as well as outputs of
climate or Earth system models from large-scale computational
platforms. However, to be able to fully realize the potential benefits
of these data sets, a number of computational challenges in
spatio-temporal data mining (STDM) need to be addressed and these
challenges are the focus of this project. Specifically, the focus is
on the development of data mining algorithms that can help answer some
of the most important questions faced by the climate and ecosystem
scientists today.